TL;DR
This week we feature a cross-post from the Deep Forest Sciences blog. Our Prithvi platform accelerates small molecule drug discovery with scientific foundation models.
Introduction
Drug discovery is a long and complex process that requires significant time and resources. The pharmaceutical industry has historically depended on an expensive trial-and-error process that tests large numbers of compounds in the lab to identify potential drug candidates. The process is expensive, costing $2.6 billion per approved drug, and time consuming, taking 10-15 years to bring a new drug to the market. The full pipeline has very low yield, with tens of thousands (or even millions or billions) of compounds tested to produce a single clinical candidate, and a 90% failure rate between entering the clinic and reaching patients.
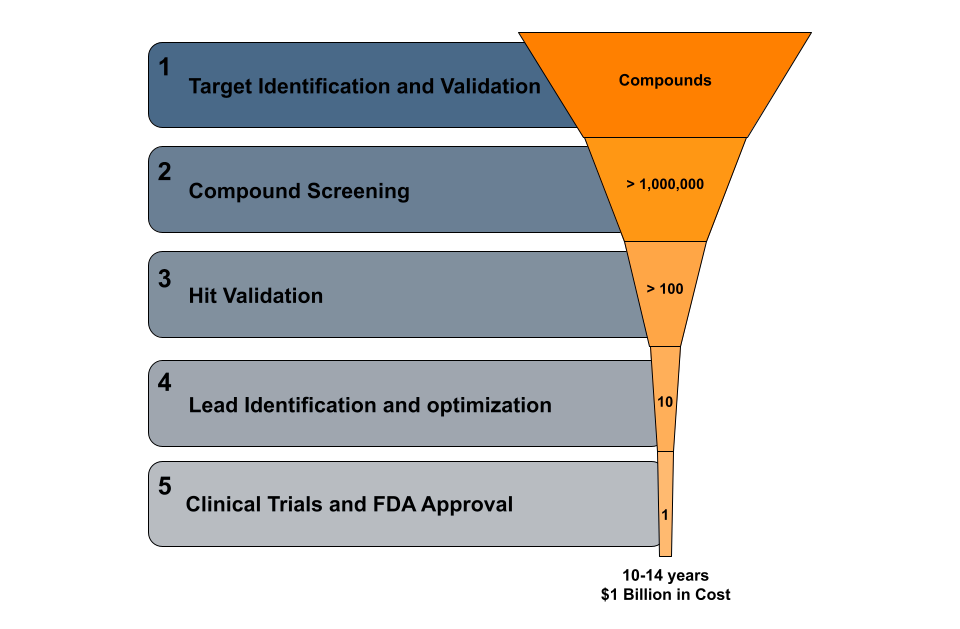
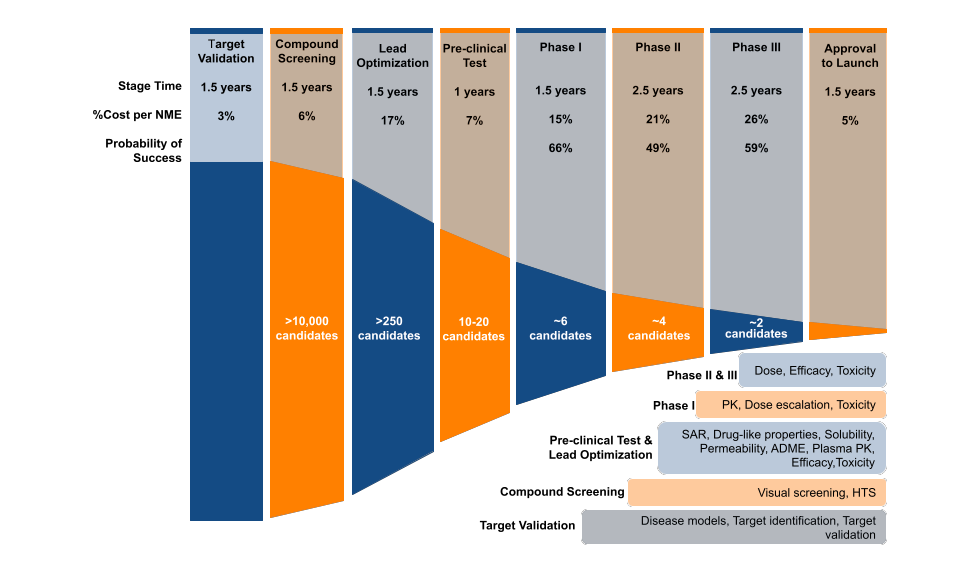
AI techniques hold out the promise of leveraging data to transform trial-and-error into guided data-driven design. By leveraging powerful machine learning models in combination with rich biological, chemical and physical priors, it ought to be possible to lower failure rates and accelerate timelines for new therapeutics. However, AI techniques remain difficult to develop and deploy. Building large machine learning models requires sophisticated algorithmic understanding, robust cloud infrastructure, and extensive engineering know-how. While the first wave of AI-driven biotech companies has built internal AI infrastructure for discovery campaigns (at high cost), their tools remain internal and specialized to particular biological or chemical systems. As a result, most biotech teams today still lack the ability to deploy and utilize effective AI infrastructure for drug discovery.
Prithvi, our new AI powered drug discovery platform, aims to close this gap and enable world-class scientific teams to use state-of-art AI systems. Built upon years of our academic and open-source research, Prithvi allows scientists to deploy powerful AI tools without having to write a single line of code.
An Introduction to Prithvi
Prithvi uses AI and scientific machine learning algorithms to accelerate the drug discovery process. More specifically, Prithvi analyzes biological and chemical datasets in order to build machine learned models that can identify small molecule hits and optimize small molecule leads. Prithvi leverages pre-trained chemical foundation models in order to lower data requirements for practical drug discovery. Prithvi can help predict the efficacy of potential compounds, identify potential side effects, and prioritize compounds to test in secondary assays.
Prithv can be used today to perform a broad range of scientific machine learning workflows to accelerate the drug discovery process. In particular, Prithvi can be used to
Perform structural analysis of targets and identify potential binding sites for a more targeted design process.
Build models based on early assay or patent data to use in large scale virtual screens for hit finding.
Construct active learning pipelines to identify and confirm high quality hits.
Suggest modifications to increase the potency, selectivity and safety of hits.
Early uses of AI in drug discovery have often been limited by low data availability. Prithvi leverages pre-trained foundation models to help systematically lower data requirements so biotech teams can start to leverage AI earlier in their design process.
Our research team is working to expand Prithvi’s applicability to every step of the drug discovery and design process. We believe that integrating Prithvi into your drug discovery pipeline can reduce your time to IND by months or more.
Behind the Scenes
Prithvi is built around a powerful scientific machine learning engine that enables our users to run sophisticated scientific calculations without having to write any new code. Prithvi features proprietary datasets and models that users can license to bootstrap their AI efforts before building in-house datasets. Prithvi has out-of-box workflows to
Run large scale virtual screens against vendor catalogs.
Construct active learning pipelines for hit finding.
Run large scaling docking analyses.
Run free energy perturbation workflows.
Fine-tune pre-trained chemical foundation models for ADMET/QSAR Modeling.
Run hyperparameter optimization to build high-quality models.
Prithvi’s scientific machine learning engine is built around a powerful cloud infrastructure that allows users to run sophisticated workflows without having to write any code. Prithvi’s no-code capabilities empower scientists to leverage their own biological and chemical expertise without having to go through an intermediary data science team. We believe that providing scientists easy access to powerful AI workflows will unlock creativity and catalyze breakthrough discoveries.
Our Science
Prithvi is built around the DeepChem ecosystem, an open source framework for molecular drug discovery and scientific machine learning. DeepChem was created by our CEO, Dr. Bharath Ramsundar, during the course of his PhD at Stanford. DeepChem offers a wide range of tools and models for scientific machine learning and deep learning, enabling researchers to predict properties, perform virtual screening, and conduct molecular featurization. DeepChem has been cited thousands of times and is used broadly in the academic and industrial drug discovery ecosystems (see this non-exhaustive listing of DeepChem-powered science). The accompanying book, Deep Learning for the Life Sciences, authored by Dr. Ramsundar and colleagues, has become a standard computational reference for the drug discovery community.
Like DataBricks, which is built around the Spark open source ecosystem created by academic research at UC Berkeley, Deep Forest Sciences is built around the DeepChem ecosystem. Prithvi productionizes and extends the DeepChem stack to handle real-world drug discovery. Prithvi leverages its cloud infrastructure to train models and gather datasets at an industrial scale not feasible with vanilla DeepChem
In particular, Deep Forest Sciences has taken a bet on the power of large scientific foundation models. The DeepChem team, led by Dr. Ramsundar, has developed the ChemBERTa and ChemBERTa-2 models, which helped demonstrate the power of chemical self-supervision to learn meaningful representations of drug-like molecules. By harnessing chemical foundation models such as ChemBERTa, Prithvi can leverage vast unlabeled datasets of chemical structures to unlock AI methods in real-world drug discovery even with limited data. Deep Forest Sciences has built both in-house cloud infrastructure and a network of collaborations with leading scientific institutions in order to train and deploy large foundation models for drug discovery and other applications.
Deep Forest Sciences has built a track record of successful partnerships with pharmaceutical companies and academic institutions to apply AI tools to real-world problems. For example, we recently partnered with Third Rock Ventures to accelerate AI-driven drug discovery for their portfolio companies using Prithvi, and have several additional partnerships in the works. These projects have validated Prithvi on real-world drug discovery campaigns.
Conclusion
Prithvi, our AI-powered scientific discovery platform, accelerates drug discovery in order to bring new treatments to patients faster. By equipping world-class biologists and chemists with world-class AI tools, Prithvi can unlock breakthrough scientific insights by enabling scientists to marry their intuition to cutting-edge AI workflows. Partnered with Deep Forest Sciences’ team of experts, Prithvi will provide your scientists the tools they need to drug challenging targets and design breakthrough therapeutics that will help save lives.
AI methods have already unlocked major scientific advances but have yet to revolutionize drug discovery. Prithvi will cross this chasm and transform how scientists discover new medicine by enabling AI-driven drug design.
Email us at partnerships@deepforestsci.com to request a demo and learn how Prithvi can help your team today!
Interesting Links from Around the Web
https://spectrum.ieee.org/high-speed-camera: Cheaper ultrafast cameras.
Feedback and Comments
Please feel free to email me directly (bharath@deepforestsci.com) with your feedback and comments!
About
Deep Into the Forest is a newsletter by Deep Forest Sciences, Inc. We’re a deep tech R&D company building Prithvi, an AI-powered scientific discovery engine for the biotech/pharma industries. Deep Forest Sciences leads the development of the open source DeepChem ecosystem. Partner with us to apply our foundational AI technologies to hard real-world problems in drug discovery. Get in touch with us at partnerships@deepforestsci.com!
Credits
Author: Bharath Ramsundar, Ph.D.
Editor: Sandya Subramanian, Ph.D.
Research and Writing: Maithili Lohakare, Rida Irfan
Awesome blog! Your blog is very informative for everyone who needs clinical trial data. Clival Database is an excellent online platform collection of clinical trial data covering 50,000 molecules from not only primary regulatory markets.
visit here- https://clival.com/