TL;DR
In this week’s post, we review some selected highlights from the 2022 AI Index Report. AI has continued to grow in both industry and academia over the last few years. While progress in computer vision and natural language processing persists, state of the art systems struggle with more complex reasoning tasks. Naive hardware parallelization may also be reaching limits, suggesting that deeper innovation will be needed for future AI scaling. Fundamental advances (or ever larger models) may be required to cross these boundaries, but the ever growing investment in AI suggests that these roadblocks may be overcome in the years to come.
Corporate and Academic Investment in AI Continues to Grow
AI continues to grow in both the industry and academy, with increased corporate spending and rising numbers of academic publications. Student enrollment in computer science in the US has also grown in tandem.
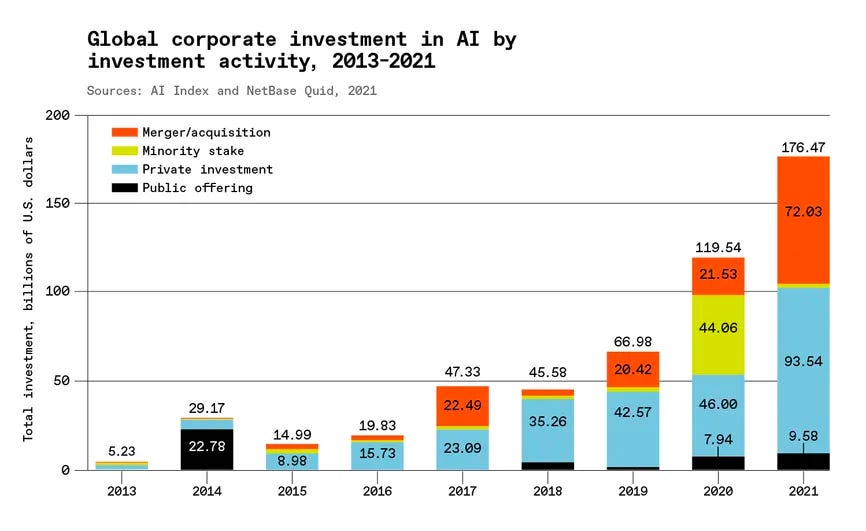
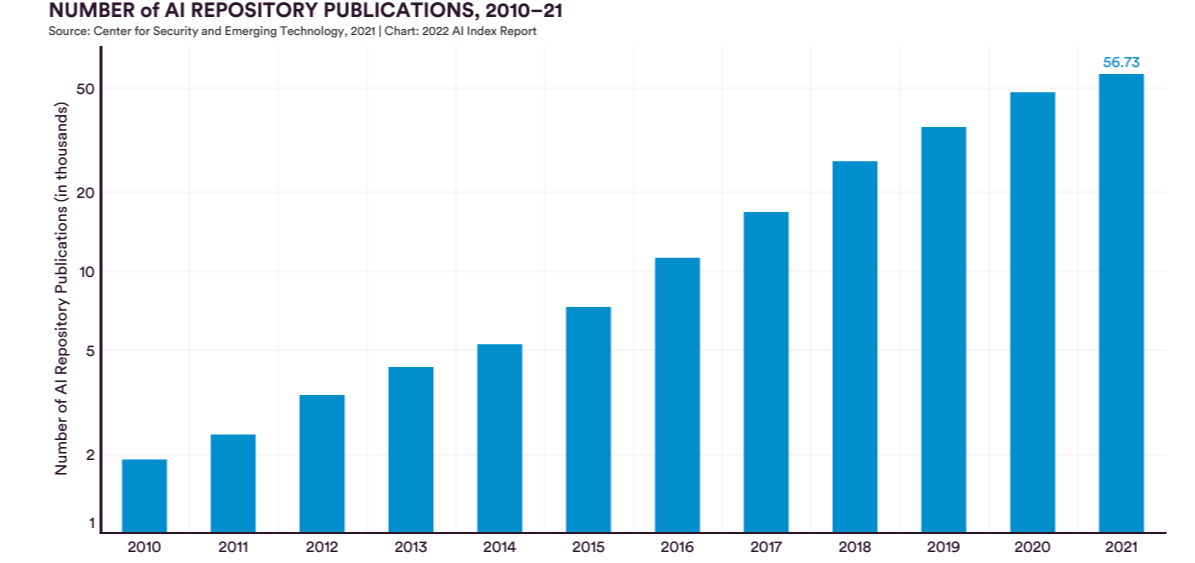
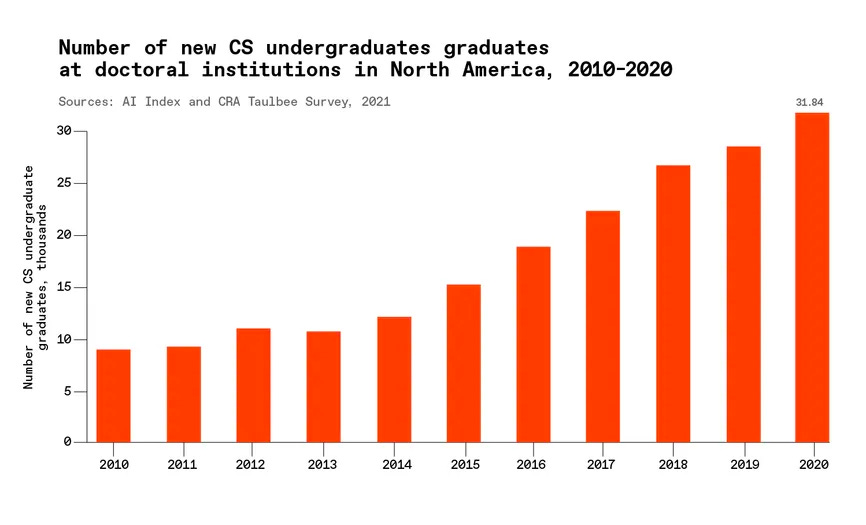
Computer Vision Progresses But More Slowly
Dramatic progress in computer vision, starting in 2012 with AlexNet, arguably triggered the deep learning revolution. This progress continues, with improving performance on complex tasks such as visual question answering, but models are plateauing in more complex visual reasoning challenges. New ideas may be required to cross the barrier between current models and human visual reasoning (but I wouldn’t rule out the possibility that a large enough vision transformer could do the trick as well.)
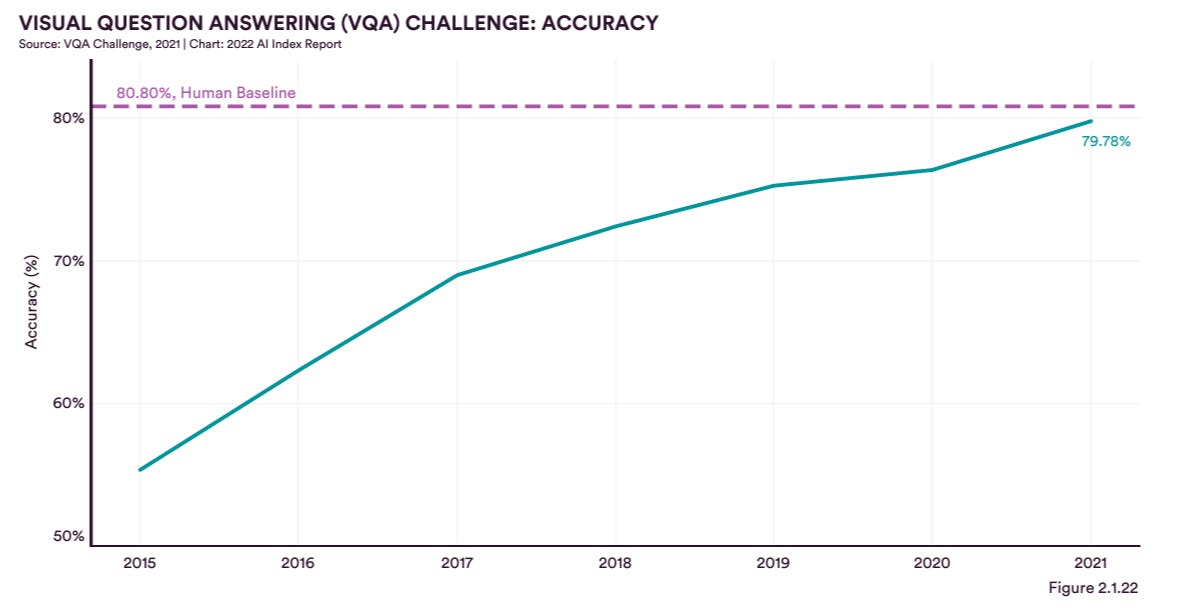
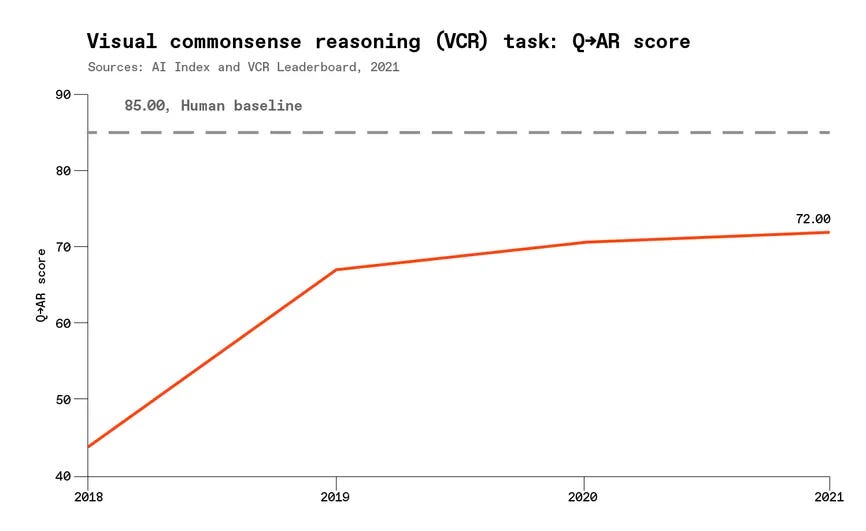
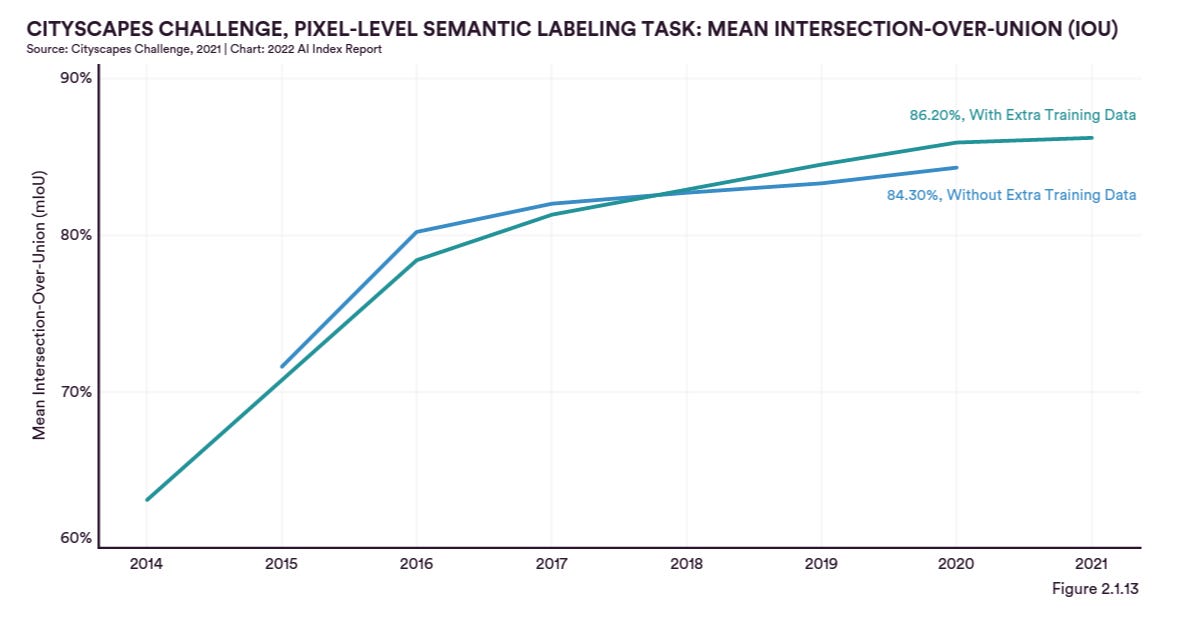
Natural Language Processing Has Made Dramatic Strides But Struggles with Reasoning
Natural language processing methods lagged behind computer vision applications of deep learning but has made dramatic strides in the last few years with the invention of transformers. However, NLP methods still struggle with more complex questions that require reasoning.
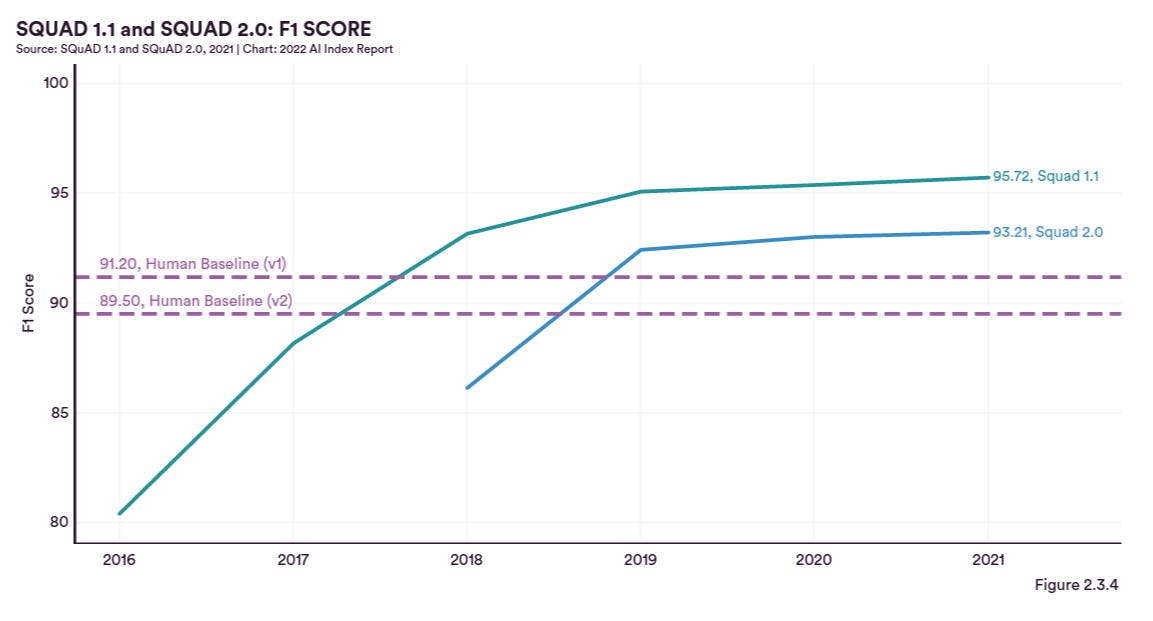
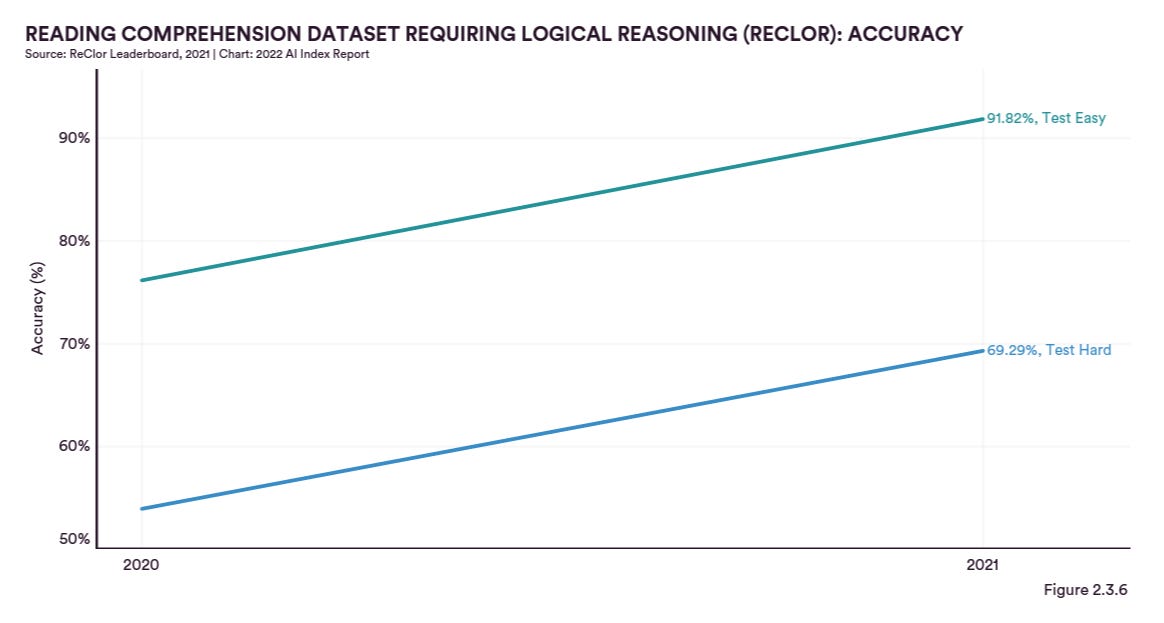
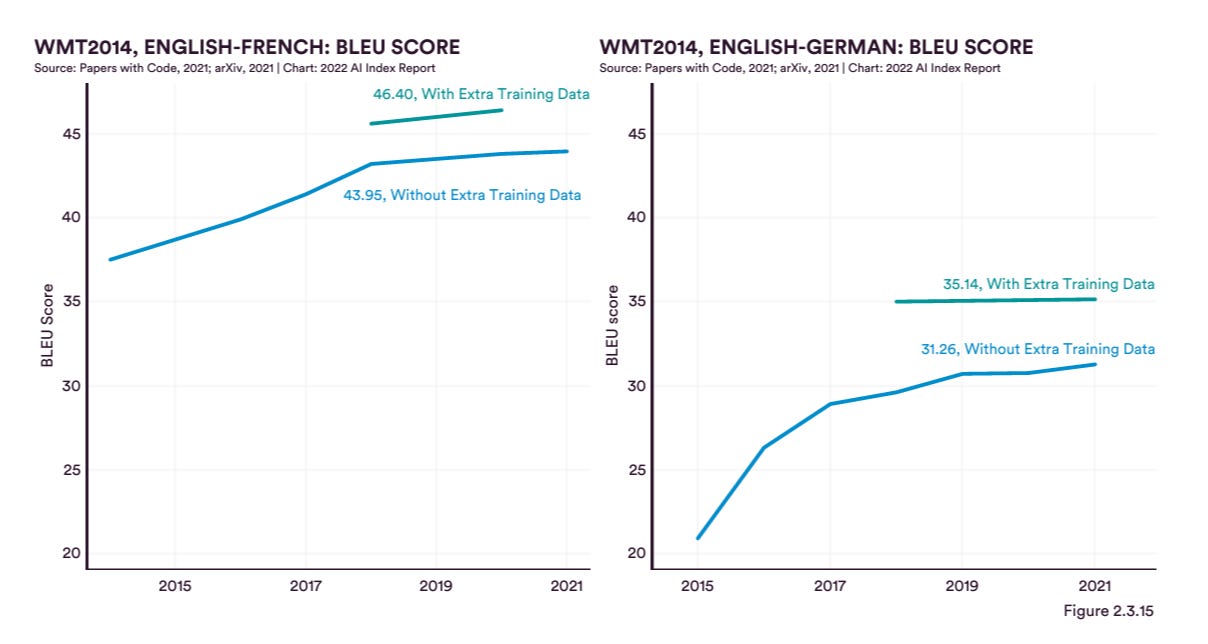
Reinforcement Learning Continues to Make Strides
Deep learning methods for reinforcement learning continue to make steady strides. While reinforcement learning has shown astounding capabilities in gameplay, real world deployments remain challenging due to the need for accurate simulation and expensive, finicky training. However, methodological advances may move reinforcement learning out of the lab and into the wild.
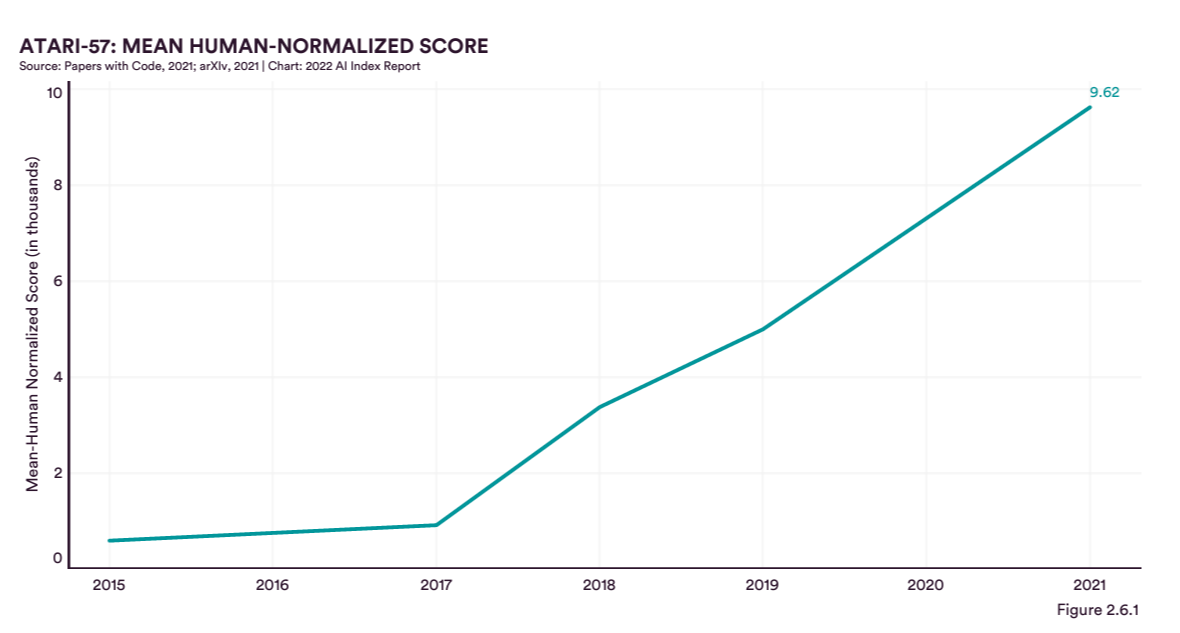
Hardware Parallelization May Be Hitting Limits
The figure below suggests that brute force hardware parallelization may be hitting communication limits. Further advances may require improving hardware accelerators at the chip level or exploiting new algorithmic advances in training capability.
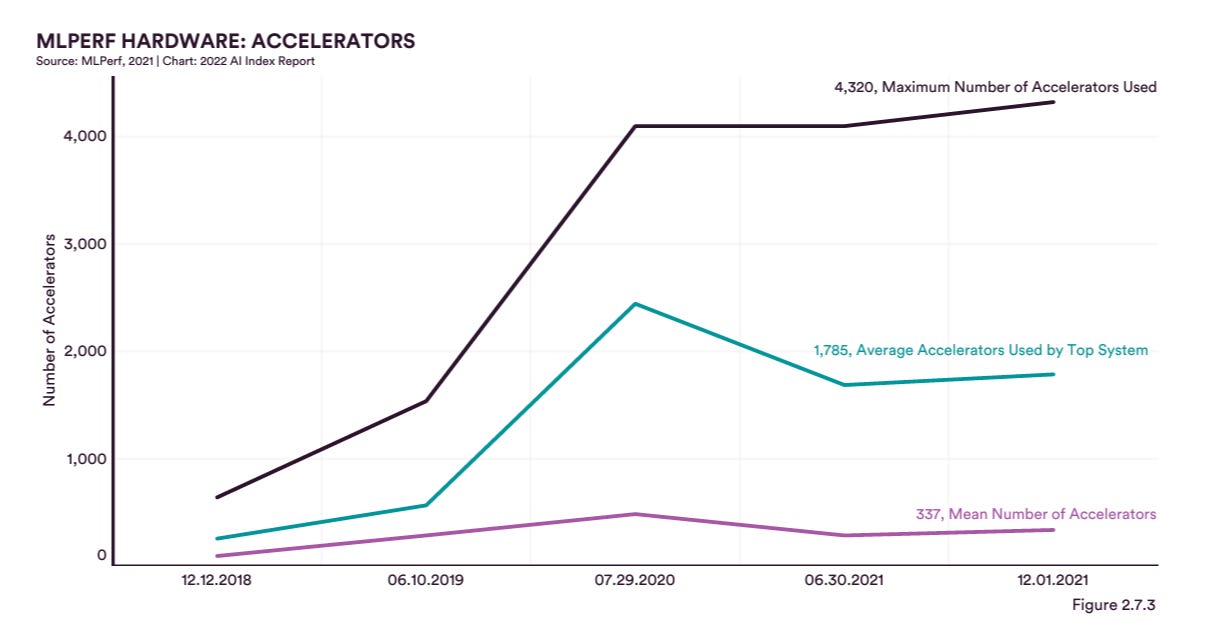
Fairness and Transparency Are Increasingly Important in AI
As AI systems are deployed more broadly, considerations of fairness, transparency and interpretability have entered center stage.
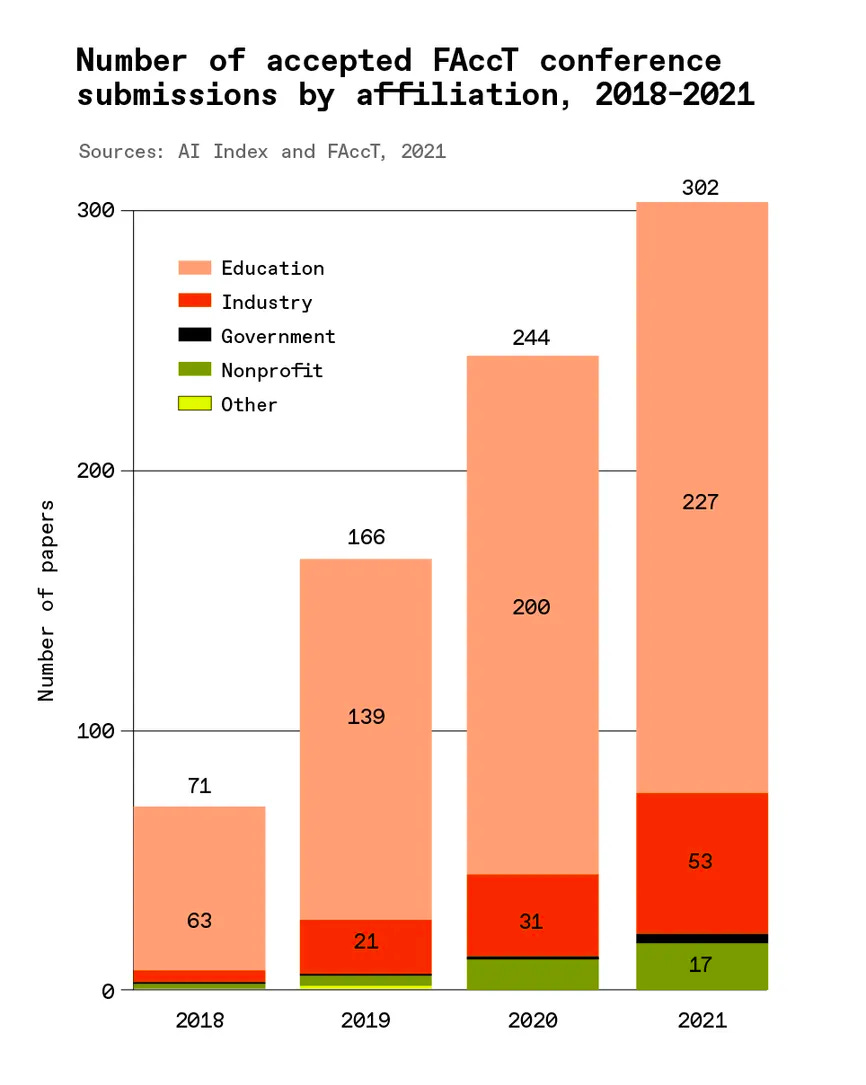
Discussion
The AI revolution has been continuing strong for a decade, starting from the dramatic advance of AlexNet for image recognition. There are signs of slowdown on multiple fronts though, with state of the art methods struggling to perform complex reasoning, and brute force hardware parallelization starting to hit a wall. However, the size of the AI industrial complex continues to grow, so I predict that progress will continue as well. My two cents is that advances in the mathematics of deep learning paired with fundamental hardware innovations could power future advances. Improved logical reasoning submodules in deep architectures will be required to enable computer vision and natural language processing systems to cross current barriers.
Weekly News Roundup
https://www.quantamagazine.org/machine-learning-reimagines-the-building-blocks-of-computing-20220315/: The field of algorithms with predictions is advancing nicely
https://fullstackeconomics.com/why-america-cant-build-big-things-any-more/: A very interesting recent essay considers reasons that America can’t quickly build big things.
https://forum.deepchem.io/t/brainstorming-gsoc-2022-topics/658: DeepChem has been accepted into Google Summer of Code 2022. Apply to work on cutting edge open source research projects!
Feedback and Comments
Please feel free to email me directly (bharath@deepforestsci.com) with your feedback and comments!
About
Deep Into the Forest is a newsletter by Deep Forest Sciences, Inc. We’re a deep tech R&D company building an AI-powered scientific discovery engine. Deep Forest Sciences leads the development of the open source DeepChem ecosystem. Partner with us to apply our foundational AI technologies to hard real-world problems. Get in touch with us at partnerships@deepforestsci.com!
Credits
Author: Bharath Ramsundar, Ph.D.
Editor: Sandya Subramanian, Ph.D.